In the realm of technology, there are occasional leaps that defy our expectations, pushing the boundaries of what we thought was possible. Quantum computing stands as one of these monumental leaps, holding the promise to revolutionize fields that have become the bedrock of our modern digital age: artificial intelligence (AI) and machine learning (ML).
Picture a world where complex calculations that once took years, even with the most powerful classical computers, are solved in a fraction of a second. Imagine AI systems that can fathom patterns within data sets of unprecedented size, providing insights that would be unattainable otherwise. Welcome to the intersection of quantum computing and AI/ML, a convergence that is reshaping our understanding of computation and problem-solving.
As we embark on this journey, we’ll delve into the exciting synergy between quantum computing and the realm of AI and ML. We’ll explore how quantum computing’s fundamental principles, like superposition and entanglement, hold the key to unlocking unprecedented computational power. The amalgamation of these two technological frontiers promises to chart new courses in everything from drug discovery to optimization challenges.
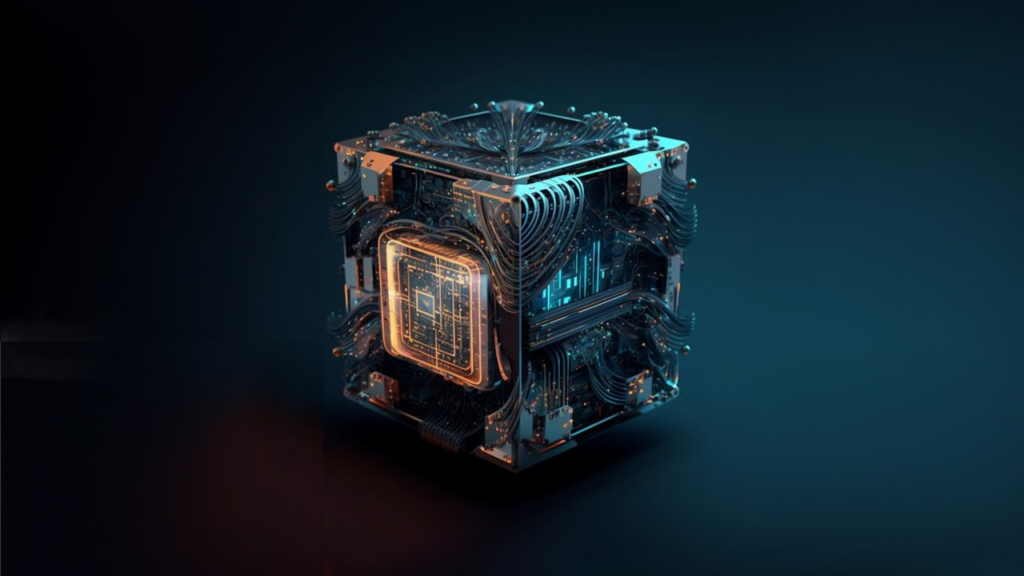
The very foundation of AI and ML rests on the ability to process and analyze data at an exponential scale. This is where quantum computing enters the stage with its unique ability to handle vast amounts of information simultaneously. The implications are profound: faster training times for AI models, novel solutions to intractable problems, and a reimagining of what’s achievable.
Join us as we embark on a journey through the uncharted landscape of quantum computing’s impact on artificial intelligence and machine learning. The future has never appeared so bright, as we stand on the precipice of a technological revolution that will leave an indelible mark on our digital world.
Contents
- 1 Understanding Quantum Computing:
- 2 The Power of Quantum Speedup:
- 3 Quantum Machine Learning (QML):
- 4 Advantages of Integrating Quantum Techniques:
- 5 Challenges of Integrating Quantum Techniques:
- 6 Potential Applications of Quantum Computing:
- 7 Current Progress and Challenges in Quantum Computing:
- 8 The Road Ahead of Quantum Computing:
- 9 Conclusion:
- 10 Additional Resources:
Understanding Quantum Computing:
Quantum computing is a groundbreaking paradigm in computation that harnesses the principles of quantum mechanics to process and manipulate information in ways that classical computers cannot. Here’s a concise overview of quantum computing and its key differences from classical computing:
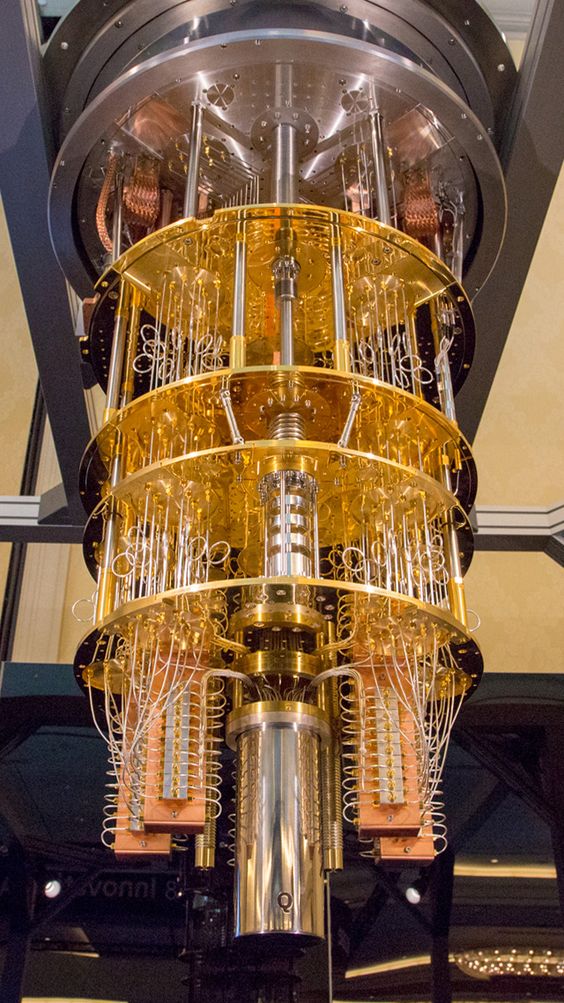
Quantum Computing: A Brief Overview
Quantum computing is based on the principles of quantum mechanics, a branch of physics that deals with the behavior of subatomic particles and their interactions. At the core of quantum computing are quantum bits, or qubits, which are the fundamental units of information. Unlike classical bits, which can only exist in states of 0 or 1, qubits can exist in a superposition of both states simultaneously. This property enables quantum computers to perform multiple calculations at once, exponentially increasing their processing power.
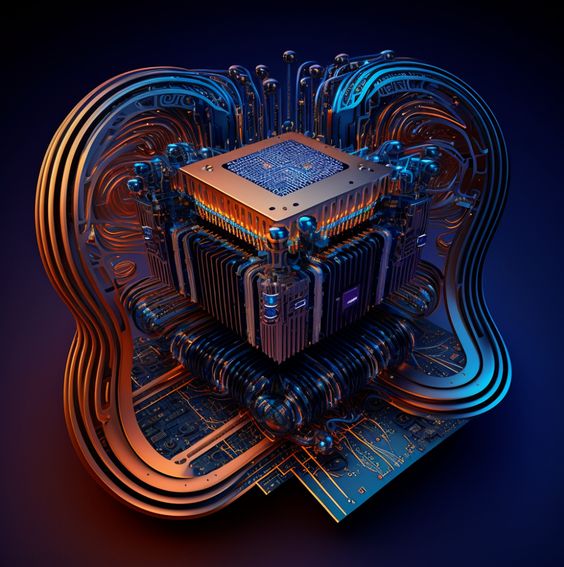
Key Differences from Classical Computing:
- Superposition: As mentioned, qubits can exist in multiple states simultaneously, allowing quantum computers to explore many possibilities at once. Classical bits can only be in one state (0 or 1) at any given time.
- Entanglement: Qubits can become entangled, meaning the state of one qubit is dependent on the state of another, even if they’re physically separated. This property enables intricate correlations and can be used for powerful computations. Classical bits cannot be entangled in the same way.
- Quantum Gates: Quantum operations are performed using quantum gates, which manipulate qubits’ states through quantum mechanical transformations. These gates are more versatile than classical logic gates, which operate on classical bits using Boolean logic.
- Measurement Uncertainty: In quantum computing, measurement collapses a qubit’s superposition to a definite state (0 or 1). However, due to the probabilistic nature of quantum mechanics, measurements can be inherently uncertain, introducing randomness into calculations.
- Quantum Parallelism: Superposition allows quantum computers to explore multiple paths simultaneously, offering a significant advantage for certain types of problems, such as optimization and searching.
- Quantum Algorithms: Quantum computers have their own algorithms that exploit their unique properties. Notable examples include Shor’s algorithm for factoring large numbers exponentially faster than classical algorithms, and Grover’s algorithm for searching unsorted databases faster.
- Error Correction Challenges: Quantum systems are sensitive to environmental disturbances, leading to errors in calculations. Quantum error correction techniques are necessary to maintain the integrity of computations, making quantum computers challenging to build and operate.
- Quantum Speedup for Specific Problems: Quantum computers offer exponential speedup for certain types of calculations, such as factoring large numbers and solving specific optimization problems. However, for many other tasks, classical computers remain more efficient.
In summary, quantum computing represents a revolutionary departure from classical computing, exploiting quantum mechanical phenomena to process information in ways that classical computers cannot. While still in its infancy, the potential applications of quantum computing are vast, particularly in tackling complex problems that have eluded classical computation.
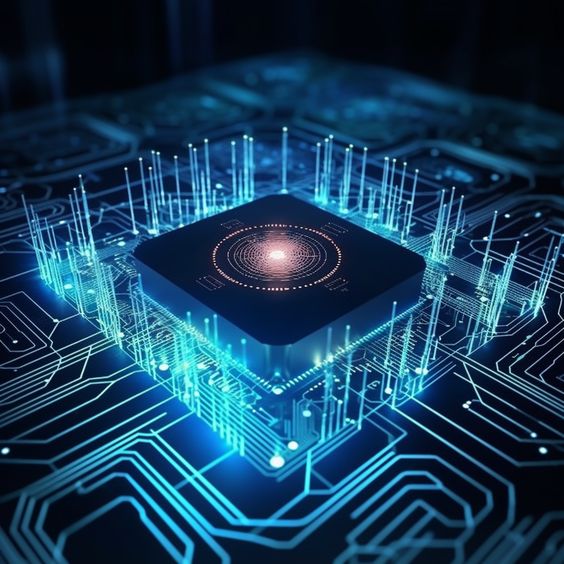
Qubits: Quantum Building Blocks
Think of qubits (quantum bits) as the building blocks of quantum computing, much like the classical bits in regular computers. But here’s the twist: while classical bits can only be 0 or 1, qubits can be 0, 1, or any combination of both at the same time. This magical property is known as “superposition.”
Superposition: The Magic of Multiple States
Imagine a spinning coin that’s both heads and tails at once, until you observe it and it settles on one side. Qubits can exist in a similar “heads and tails” state, simultaneously holding different possibilities. This ability to be in multiple states at once is what makes quantum computing so powerful, as it lets quantum computers explore many options simultaneously.
Entanglement: Quantum Connection
Entanglement is like a quantum connection that exists between certain qubits. Imagine having two linked coins: no matter how far apart they are, if you flip one, the other instantly matches its state. Qubits can become entangled, meaning the state of one qubit instantly affects the state of another, even if they’re far apart. This mysterious connection is what allows quantum computers to work on complex problems with remarkable efficiency.
Quantum Gates: The Quantum Builders
In classical computers, logic gates perform operations on bits, like adding or comparing. Quantum computers have their own special version called quantum gates. These gates apply transformations to qubits, changing their superposition and entanglement properties. Quantum gates are like tools that sculpt qubits into the desired states, allowing for complex calculations.
Putting It Together: Quantum Potential
Imagine you’re solving a maze. In a classical computer, you’d explore one path at a time. In a quantum computer, you’d explore all paths simultaneously, thanks to superposition. Additionally, if you have entangled qubits, it’s like having multiple “you”s exploring the maze at once and sharing information instantly. This quantum parallelism gives quantum computers the potential for solving certain problems much faster than classical computers.
Remember, quantum computing isn’t just about processing power; it’s about using these peculiar quantum behaviors to tackle challenges that would be impossible or incredibly slow with classical computers. While quantum computing is still evolving and has its share of challenges (like handling errors), it holds the promise to unlock new frontiers in science, technology, and problem-solving that were once thought to be beyond reach.
The Power of Quantum Speedup:
Certainly, quantum computers have the potential to achieve exponential speedup for specific types of calculations when compared to classical computers. This phenomenon is a result of the unique properties of qubits, such as superposition and entanglement. Let’s explore how this speedup works and what it means for various computational tasks:
Exponential Speedup: Unleashing Quantum Power
Imagine you’re looking for a specific item in a large library. Classical computers would search through books one by one, taking time proportional to the number of books. Quantum computers, however, can explore multiple options simultaneously due to superposition. For certain tasks, this leads to an exponential speedup, meaning the time needed to complete the task decreases significantly as the problem size increases.
Factoring Large Numbers: Shor’s Algorithm
One of the most famous examples of quantum speedup is Shor’s algorithm for factoring large numbers into their prime components. In classical computers, this task becomes incredibly slow as the number’s size increases. Shor’s algorithm, on the other hand, can factor large numbers exponentially faster using quantum computers. This has implications for cryptography, as many encryption methods rely on the difficulty of factoring large numbers.
Quantum Simulation: Exploring Complex Systems
Another area where quantum computers excel is simulating quantum systems, which is challenging for classical computers due to the immense complexity of quantum mechanics. Quantum computers can simulate quantum systems more efficiently, which has applications in fields like materials science, chemistry, and drug discovery. They can simulate molecular interactions and properties, leading to the discovery of new materials and drugs.
Optimization: Solving Complex Problems
Many real-world problems involve optimization, such as finding the most efficient route for deliveries or the best arrangement for components. Quantum computers can explore different solutions in parallel, providing faster results for certain optimization problems. This has applications in logistics, supply chain management, and resource allocation.
Machine Learning and Data Analysis: Quantum Speedup
Machine learning and data analysis tasks often involve complex calculations, such as training large models or analyzing vast datasets. Quantum computers could speed up these processes by processing multiple possibilities simultaneously. Quantum machine learning algorithms could also discover patterns and insights more quickly than classical methods.
Limitations and Challenges: Not a Universal Solution
While quantum speedup is a remarkable advantage, it’s essential to note that quantum computers aren’t universally faster for all tasks. They’re highly specialized and excel at problems that align with their unique properties. Additionally, quantum computers are currently in an experimental stage, facing challenges such as qubit stability, error correction, and scaling.
In conclusion, quantum computers offer the potential for exponential speedup for specific calculations that are difficult or slow for classical computers. This potential opens doors to solving problems that were previously beyond reach due to computational complexity. As quantum technology advances and researchers tackle the challenges, we may witness breakthroughs that redefine the possibilities of computing and problem-solving.
Optimization problems and complex simulations are integral components of fields like artificial intelligence (AI) and machine learning (ML). These problems involve searching for the best solution among a vast number of possibilities, and they can become computationally intensive as the complexity of the problem or the size of the data increases. Quantum speedup has the potential to provide significant benefits in these areas. Let’s explore why:
Optimization Problems:
Optimization problems involve finding the best solution from a set of possible solutions, often with the goal of maximizing or minimizing a specific objective function. In AI and ML, optimization is at the core of training machine learning models, fine-tuning parameters, and solving complex decision-making scenarios.
- Parallel Exploration: Quantum computers can explore multiple possibilities simultaneously due to the concept of superposition. In optimization, this means that a quantum computer can simultaneously evaluate different potential solutions. Classical computers evaluate solutions sequentially, which becomes time-consuming for complex problems. Quantum computers can potentially identify optimal solutions more quickly by exploring multiple paths at once.
- Exponential Speedup: Some optimization problems become increasingly difficult as the number of variables or constraints grows. Classical algorithms often experience exponential time complexity in such cases. Quantum computers, with their exponential speedup potential, could provide solutions to these problems in significantly less time than classical methods.
- Global Search: Quantum computers could aid in finding global optima in complex optimization landscapes. Classical optimization methods can sometimes get trapped in local optima, missing the best solution. Quantum computers’ ability to explore multiple options in parallel might help them escape local optima and find more optimal solutions.
Complex Simulations:
In AI and ML, complex simulations involve modeling and simulating intricate real-world systems to gain insights or make predictions. These simulations are resource-intensive and can take a long time to complete. Quantum computing can offer advantages in simulating quantum systems, materials, and chemical reactions, which are relevant to AI and ML applications.
- Quantum System Simulation: Quantum computers excel at simulating quantum systems that are notoriously difficult for classical computers to simulate accurately. These simulations are valuable for developing new materials, understanding molecular interactions, and advancing fields like quantum chemistry.
- Time Complexity Reduction: Quantum simulations of quantum systems can achieve polynomial time complexity, compared to classical methods that often have exponential time complexity. This reduction in time complexity means that complex simulations could be completed more quickly on quantum computers.
- Molecular Modeling: Quantum computers’ ability to simulate molecular interactions could lead to faster drug discovery and materials research. They could simulate chemical reactions and molecular properties with higher accuracy and speed than classical methods.
In summary, optimization problems and complex simulations in AI and ML stand to benefit greatly from quantum speedup. Quantum computers have the potential to explore multiple options simultaneously, solve problems with exponential speedup, and simulate quantum systems more efficiently. While quantum computers are still in the early stages of development, their unique capabilities hold promise for transforming how we approach optimization challenges and complex simulations in various scientific and technological domains.
Quantum Machine Learning (QML):
Unleashing Quantum Potential in Machine Learning
In the ever-evolving landscape of technology, new frontiers are constantly emerging. One such frontier that has captured the imagination of scientists and researchers alike is the intersection of two groundbreaking fields: Quantum Computing and Machine Learning. The result of this convergence is Quantum Machine Learning (QML), a paradigm that marries the power of quantum mechanics with the sophistication of machine learning.
Quantum Machine Learning: The Quantum Leap in AI
Quantum Machine Learning (QML) represents a fusion of quantum computing principles and traditional machine learning techniques. At its core, QML harnesses the remarkable properties of qubits, the building blocks of quantum computation, to enhance the capabilities of classical machine learning algorithms.
The Quantum Advantage: Enhancing Traditional Algorithms
Imagine enhancing the brainpower of machine learning algorithms by imbuing them with the uncanny properties of quantum physics. Qubits, unlike classical bits, exist in multiple states simultaneously, a phenomenon known as superposition. Additionally, qubits can become entangled, enabling a level of parallelism and correlation that classical bits can’t achieve.
In the realm of QML, these properties translate into a unique edge: the ability to process and analyze vast amounts of data in parallel, leading to exponential speedup for certain tasks. Traditional machine learning algorithms that involve optimization, pattern recognition, and data analysis can be turbocharged by leveraging quantum parallelism and entanglement.
QML in Action: Shaping the Future
Consider a scenario where complex optimization problems in machine learning, such as hyperparameter tuning for neural networks, can be tackled with unprecedented efficiency. Or envision quantum computers performing intricate data analysis with enhanced precision and speed, revealing hidden insights within massive datasets.
Quantum Machine Learning doesn’t aim to replace classical machine learning methods; rather, it seeks to complement and extend their capabilities. By exploiting the quantum realm’s unique properties, QML opens the door to solving problems that were once considered computationally infeasible.
The Road Ahead: Unveiling Quantum’s Potential
As quantum computing technology matures, Quantum Machine Learning promises to transform the landscape of AI and machine learning. Researchers are exploring new algorithms and techniques that leverage quantum speedup, enhancing our ability to solve complex problems across diverse domains.
In the chapters to come, we’ll delve deeper into the inner workings of Quantum Machine Learning. We’ll explore specific algorithms, applications, and the challenges that lie ahead as we strive to unlock the full potential of quantum-enhanced machine learning. Welcome to the world of Quantum Machine Learning, where quantum mechanics meets the art of machine intelligence.
Advantages of Integrating Quantum Techniques:
- Exponential Speedup: Quantum computers have the potential to provide exponential speedup for certain computations, such as solving linear systems of equations. By integrating quantum techniques, classical ML algorithms that rely on these computations can become significantly faster.
- Enhanced Optimization: Quantum parallelism and entanglement can revolutionize optimization algorithms. Traditional optimization problems in ML, like training neural networks or finding optimal hyperparameters, could see faster convergence and improved results.
- Complex Data Analysis: Quantum computers excel at handling large-scale data analysis due to their ability to process multiple data points simultaneously. This is advantageous for ML tasks that involve analyzing massive datasets, extracting patterns, and identifying anomalies.
- Feature Space Expansion: Quantum techniques can be used to create new features and representations of data, potentially enabling more efficient and accurate learning models. This can improve the performance of ML algorithms, especially in cases where feature engineering is crucial.
- Solving Quantum-Inspired Problems: Quantum techniques might find applications in problems that exhibit quantum-like behavior, even if they’re not explicitly quantum. These problems could be better addressed using hybrid quantum-classical approaches.
Challenges of Integrating Quantum Techniques:
- Quantum Hardware Limitations: Quantum computers are still in their infancy, with limited qubits and susceptibility to noise and errors. These constraints make it challenging to perform complex computations reliably and at scale.
- Qubit Interactions: Effective quantum computation often relies on qubit-qubit interactions. Designing quantum algorithms that harness these interactions while minimizing errors is complex and requires expertise in both quantum and classical computing.
- Hybrid Approaches: Integrating quantum techniques into classical ML algorithms often involves creating hybrid quantum-classical systems. Developing algorithms that combine both paradigms effectively requires careful consideration of how they interact and complement each other.
- Quantum Error Correction: Error rates in quantum computers are high, necessitating error correction techniques. Integrating these error correction mechanisms into ML algorithms can be challenging and might negate some of the computational benefits.
- Algorithm Adaptation: Classical ML algorithms may need to be adapted or rethought to take full advantage of quantum enhancements. This requires expertise in both classical ML and quantum computing.
- Expertise Gap: The field of quantum computing is specialized, and experts who understand both quantum mechanics and ML are relatively rare. Collaborations between quantum physicists and machine learning practitioners are essential for successful integration.
In conclusion, integrating quantum techniques into classical ML algorithms presents exciting possibilities for solving problems faster and more efficiently. However, it’s essential to navigate the challenges posed by the current state of quantum technology, hardware limitations, and algorithmic complexities. As quantum computing matures and research progresses, hybrid quantum-classical approaches could lead to groundbreaking advancements in the realm of machine learning.
Potential Applications of Quantum Computing:
1. Faster Training of Complex Machine Learning Models:
Quantum computers can dramatically accelerate the training process of complex machine learning models. Training deep neural networks involves iterative optimization, a task that can take a significant amount of time on classical computers. Quantum optimization algorithms could lead to faster convergence and reduced training times, enabling the exploration of larger and more sophisticated models.
2. Improved Optimization Techniques for AI Tasks:
Optimization is fundamental to AI tasks like feature selection, parameter tuning, and model optimization. Quantum optimization algorithms, such as Quantum Approximate Optimization Algorithm (QAOA), can potentially find optimal solutions faster than classical algorithms. This could lead to more accurate models and improved AI system performance.
3. Enhanced Data Analysis for Pattern Recognition and Anomaly Detection:
Quantum computers can process and analyze vast datasets in parallel, making them well-suited for pattern recognition and anomaly detection. In fields like image analysis, speech recognition, and fraud detection, quantum-enhanced algorithms could unveil hidden patterns and anomalies that might have been overlooked with classical methods.
4. Solving Previously Intractable Problems:
Quantum computing’s most exciting promise lies in solving problems that are computationally intractable for classical computers. In fields like drug discovery, cryptography, and materials science, quantum computers could revolutionize research:
- Drug Discovery: Quantum simulations could accurately model molecular interactions, leading to the discovery of new drugs and therapies with unprecedented precision.
- Cryptography: Quantum computers could break classical cryptographic methods, but they could also enable quantum cryptography techniques that provide unbreakable security.
- Materials Science: Quantum simulations can predict the properties of complex materials, leading to innovations in energy storage, electronics, and materials design.
5. Quantum-Inspired Machine Learning:
Quantum techniques can inspire new machine learning algorithms even in classical settings. Quantum-inspired algorithms, like Quantum Boltzmann Machines, might unlock novel ways to tackle optimization and unsupervised learning problems, expanding the ML toolkit.
6. Hybrid Quantum-Classical Systems:
Many real-world problems require a combination of quantum and classical approaches. Hybrid quantum-classical systems allow for the best of both worlds. Quantum computers can be used for specific subproblems, while classical computers handle the rest, offering a pragmatic solution to harness quantum advantages within the current limitations of quantum hardware.
As quantum computing technology advances, its impact on AI and ML is likely to become more pronounced. Researchers and practitioners are exploring innovative algorithms and applications that leverage quantum speedup and unique quantum properties to push the boundaries of what’s possible in AI, machine learning, and various scientific disciplines.
Current Progress and Challenges in Quantum Computing:
Recent Advancements and Collaborations:
- Quantum Hardware Improvements: Quantum computing companies like IBM, Google, and Rigetti have made strides in improving the stability and coherence of qubits. This has led to advancements in quantum volume, a metric that combines qubit quality and error rates to measure quantum processor capabilities.
- Quantum Machine Learning Algorithms: Researchers have been developing quantum algorithms that directly integrate with machine learning tasks. For instance, Variational Quantum Eigensolver (VQE) and Quantum Neural Networks (QNNs) are being explored for quantum-enhanced optimization and classification tasks.
- Quantum Software Platforms: Companies are building user-friendly quantum development platforms that allow researchers to experiment with quantum algorithms and explore their applications in machine learning. These platforms help bridge the gap between quantum and classical computing expertise.
- Hybrid Quantum-Classical Approaches: Collaboration between quantum computing and AI/ML researchers has led to hybrid approaches where quantum computers handle specific subtasks within a larger classical machine learning pipeline. This synergy capitalizes on quantum strengths while accommodating current hardware limitations.
- Quantum-Inspired Algorithms: AI/ML researchers are also developing quantum-inspired classical algorithms that emulate some of the behavior and benefits of quantum computing. These algorithms aim to capture quantum speedup without requiring actual quantum hardware.
Current Limitations and Challenges:
- Qubit Fragility: Qubits are highly sensitive to their environment and external disturbances. This makes maintaining qubit coherence and reducing noise a significant challenge, affecting the overall accuracy and reliability of quantum computations.
- Error Correction: Quantum computations are prone to errors due to noise and other factors. Developing effective error correction techniques, which are essential for scaling up quantum computers, is an ongoing challenge.
- Quantum Decoherence: Qubits lose their quantum properties over time due to a phenomenon called decoherence. This limits the time available for performing computations and requires advanced error mitigation techniques.
- Limited Qubit Count: Quantum computers currently have a limited number of qubits, which constrains the complexity of problems they can handle. Achieving the necessary qubit scale for certain quantum algorithms remains a hurdle.
- Scalability: Scaling up quantum computers while maintaining qubit quality and reducing error rates is a complex engineering challenge. As qubit count increases, the difficulties of qubit-qubit interactions and maintaining coherence become more pronounced.
- Lack of Quantum-Ready Data: Quantum algorithms require quantum-ready data, which might be different from classical data. Preparing data for quantum computation and adapting existing ML datasets for quantum use is an area that needs further research.
- Interdisciplinary Expertise: Successful quantum-enhanced AI/ML research requires expertise in both quantum physics and machine learning. Bridging this interdisciplinary gap is essential for making the most of the collaboration.
In conclusion, recent advancements in quantum computing, coupled with collaborations between quantum computing and AI/ML research communities, are opening up new possibilities for solving complex problems. However, the current limitations and challenges, such as qubit fragility, error correction, and scalability, remind us that quantum computing is still a frontier technology. Progress is being made, but researchers continue to work diligently to address these challenges and unlock the full potential of quantum-enhanced AI and machine learning.
The Road Ahead of Quantum Computing:
Hybrid Quantum-Classical Systems: Bridging the Gap:
Hybrid quantum-classical systems are a powerful approach that leverages the strengths of both quantum and classical computing. These systems acknowledge the current limitations of quantum hardware while capitalizing on its unique capabilities. Here’s how they work and their significance:
- Quantum Acceleration: Quantum processors can be used for specific subtasks that can benefit from quantum speedup, such as optimization or certain simulations. These tasks are embedded within a larger classical computation, thus harnessing quantum advantages without relying entirely on error-prone quantum operations.
- Error Mitigation: Hybrid systems can implement error correction and mitigation techniques from classical computing to improve the reliability of quantum computations. Classical algorithms can detect and correct quantum errors, enhancing the overall accuracy of results.
- Scalability: Hybrid systems can handle problems that currently exceed the capabilities of quantum hardware alone. As quantum hardware improves, these systems can adapt and incorporate more quantum processes, gradually transitioning to more purely quantum solutions.
- Problem-Specific Solutions: Hybrid systems allow researchers to tackle real-world problems with quantum-enhanced solutions. This approach is practical since quantum computers might not need to perform every step of a computation to provide valuable insights or speedup.
Long-Term Impact of Quantum Computing on Artificial Intelligence:
The long-term impact of quantum computing on artificial intelligence is a topic of immense excitement and speculation. While predicting the exact outcomes is challenging, we can envision several possibilities:
- Revolutionized Training and Modeling: Quantum speedup could revolutionize the training of complex AI models. Faster convergence and more efficient optimization could lead to the development of deeper, more accurate models.
- Transformed Data Analysis: Quantum computers could dramatically accelerate data analysis tasks. Pattern recognition, anomaly detection, and large-scale data processing could become substantially more efficient and accurate.
- Advances in Optimization: Quantum computing’s optimization capabilities could lead to more effective AI systems that can optimize resources, make better decisions, and navigate complex decision landscapes more efficiently.
- Unveiling New Insights: Quantum-enhanced simulations could lead to the discovery of new scientific insights and breakthroughs, translating into innovative AI algorithms and applications.
- Quantum-Inspired Algorithms: Quantum techniques could inspire new algorithms that harness some of the quantum advantages even in classical settings, expanding the ML toolkit.
- Quantum-Resilient Security: Quantum cryptography and security solutions might become essential components in ensuring the integrity and privacy of AI systems in a quantum-powered world.
- Unlocking New Frontiers: The intersection of quantum computing and AI might lead to novel discoveries and innovations that we can’t yet imagine, opening up entirely new directions for technological development.
In essence, while quantum computing is still in its infancy, its potential impact on artificial intelligence is profound. Quantum speedup, enhanced optimization, and advanced simulations could reshape how we develop AI systems, process data, and solve complex problems. The long-term future likely holds a harmonious coexistence of classical and quantum techniques, with hybrid systems and quantum-enhanced algorithms revolutionizing the AI landscape in unprecedented ways.
Conclusion:
Summary: Unlocking the Future – Quantum Computing’s Transformation of AI and ML
In the ever-evolving world of technology, a transformative partnership is emerging at the crossroads of quantum computing and artificial intelligence (AI) and machine learning (ML). Quantum computing’s unique properties, such as superposition and entanglement, hold the key to a new era of possibilities for AI and ML. Here’s a recap of the transformative potential we’ve explored:
- Quantum Speedup: Quantum computing offers exponential speedup for specific calculations, solving complex problems that were previously impractical or time-consuming for classical computers.
- Optimization and Simulations: Quantum computing excels in optimization problems and complex simulations, enabling faster convergence and insights in fields like materials science, drug discovery, and AI tasks.
- Quantum Machine Learning: The integration of quantum techniques into classical ML algorithms enhances optimization, data analysis, and model training. This Quantum Machine Learning (QML) frontier promises unprecedented gains in efficiency.
- Hybrid Systems: The synergy between quantum and classical computing through hybrid systems addresses current quantum limitations while harnessing quantum advantages to solve real-world problems.
- Advancements and Challenges: Recent advancements in quantum hardware, algorithms, and collaborations with AI/ML communities are paving the way for groundbreaking innovations. However, challenges like qubit fragility and error correction remain to be overcome.
Embrace the Future: Stay Informed and Engaged
As quantum computing continues to mature, its convergence with AI and ML holds tremendous promise for reshaping the technological landscape. We encourage you to stay updated on advancements in both quantum computing and AI/ML, as they are poised to shape the future of technology in profound ways. Whether you’re a scientist, researcher, or enthusiast, keeping abreast of developments in these fields will equip you to be part of the transformative journey ahead. Quantum-powered AI and ML have the potential to unlock discoveries, insights, and capabilities that can redefine our understanding of computation, science, and problem-solving. The future is unfolding at the nexus of quantum and intelligence – don’t miss your chance to be part of it.
Additional Resources:
Here are some resources that you can explore to delve deeper into the subject of quantum computing, AI, and their intersection:
Quantum Computing:
- IBM Quantum Experience: IBM’s quantum computing platform offers resources, tutorials, and hands-on experience with quantum computers.
- Quantum Computing Report: A comprehensive source for news, updates, and analysis related to the field of quantum computing.
- arXiv Quantum Physics: Research papers on quantum physics and computing available on arXiv.
Quantum Machine Learning:
- Quantum Machine Learning: A special issue of Quantum – a journal for quantum science and technology – dedicated to quantum machine learning research.
- Quantum Machine Learning Research Papers: Quantum journal’s collection of research papers on quantum machine learning.
Hybrid Quantum-Classical Systems:
- Hybrid Quantum-Classical Algorithms: A research paper discussing hybrid quantum-classical algorithms and their applications.
- Hybrid Quantum-Classical Neural Networks: A paper exploring hybrid quantum-classical neural networks for machine learning tasks.
Advancements and Collaborations:
- Quantum AI Lab – Google: Google’s Quantum AI Lab focuses on exploring quantum algorithms for machine learning and optimization.
- Microsoft Quantum Computing: Microsoft’s quantum computing initiative, including research and resources.
- D-Wave Quantum Computing: D-Wave Systems focuses on quantum annealing and its applications, including optimization problems.
Articles and Overviews:
- Quantum Computing for the Very Curious: A detailed guide to quantum computing concepts and principles.
- Quantum Machine Learning: What You Need to Know: An article providing an overview of quantum machine learning and its potential applications.
Remember to explore academic databases like PubMed, IEEE Xplore, and ACM Digital Library for scholarly articles on quantum computing and its intersections with AI and machine learning. As the field is rapidly evolving, staying engaged with the latest research, publications, and news will provide you with a comprehensive understanding of the subject.